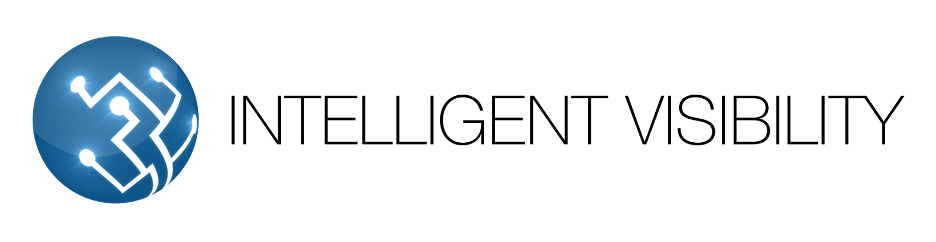
AIOps for Intelligent IT Infrastructure Management
Beyond the Noise: Harnessing AIOps for Intelligent IT Infrastructure Management
Introduction: Taming IT Chaos – The Rise of AIOps for Infrastructure
Modern IT infrastructure teams operate under immense pressure. They are tasked with supporting rapid innovation, ensuring flawless application performance, and managing increasingly complex, distributed environments that span on-premises data centers, multiple public clouds, edge locations, and containerized workloads. Simultaneously, these teams are often expected to achieve these demanding goals with constrained resources, embodying the mantra of "doing more with less". This operational environment, characterized by escalating complexity and relentless demands, has pushed traditional IT management approaches to their breaking point.
Enter AIOps (Artificial Intelligence for IT Operations). Defined by Gartner as technology that "combines big data and machine learning to automate IT operations processes, including event correlation, anomaly detection and causality determination" 4, AIOps represents a fundamental shift in how IT infrastructure is managed. It moves beyond the limitations of manual intervention and static rule-based systems, leveraging artificial intelligence to make sense of the vast amounts of data generated by modern IT environments. While often discussed in the context of networking, the true potential of AIOps is realized when applied holistically across the entire IT infrastructure stack – encompassing servers, storage, virtualization platforms, cloud services, databases, middleware, and applications. This comprehensive approach provides the unified visibility necessary to manage interconnected systems effectively.
The core promise of AIOps is transformative: it aims to shift IT operations from a reactive, "firefighting" mode to a proactive, predictive, and increasingly automated paradigm. By intelligently analyzing operational data, AIOps platforms can identify potential issues before they impact users, accelerate the resolution of incidents when they do occur, and automate routine tasks, freeing up valuable human expertise for more strategic initiatives. Ultimately, this operational evolution translates directly into tangible business value through enhanced system reliability, improved operational efficiency, increased agility, and better overall user and customer experiences. Navigating this transition requires expertise and strategic partnership, guiding organizations toward intelligent operations. The adoption of AIOps is therefore not merely a technological upgrade; it is increasingly becoming a strategic imperative for organizations seeking to maintain operational stability and competitive advantage in a complex digital world. The pressures driving this shift – overwhelming data volumes, system complexity, and the business impact of downtime – necessitate a more intelligent approach to IT infrastructure management.
The Breaking Point: Why Traditional IT Operations Can't Keep Pace
The limitations of traditional IT operations methodologies become starkly apparent when confronted with the realities of modern digital infrastructure. Several key challenges consistently overwhelm legacy tools and manual processes:
The Data Deluge: Today's IT environments generate an unprecedented volume, velocity, and variety of telemetry data – logs, metrics, traces, events – from an ever-expanding array of sources including servers, network devices, cloud platforms, containers, microservices, and IoT devices. Gartner highlights that 85% of IT leaders identify this sheer volume of data as their most significant challenge. Compounding this is the variety of data formats and structures, making aggregation and analysis incredibly complex using traditional means. Manual analysis is simply no longer feasible at this scale.
Hybrid Complexity & Ephemeral Environments: Managing infrastructure spread across on-premises data centers, multiple public clouds, and edge locations introduces significant complexity and often results in visibility gaps. Dynamic and ephemeral components, such as containers and serverless functions, further complicate monitoring and management, as resources appear and disappear rapidly, making static configurations obsolete. Traditional tools often struggle to provide a unified view across these disparate and transient environments.
Tool Sprawl & Siloed Monitoring: Organizations frequently accumulate a multitude of monitoring tools, each focused on a specific domain (e.g., network performance, server health, application monitoring, cloud resource usage). While often necessary historically, this "tool sprawl" leads to fragmented visibility, operational silos, redundant data collection efforts, and a lack of integrated analysis. This fragmentation prevents a holistic understanding of system health and hinders effective troubleshooting. Tool sprawl is thus both a symptom of reactive problem-solving and a direct cause of ongoing operational inefficiency, creating a cycle that AIOps seeks to break by unifying data and analysis.
Alert Fatigue & Noise: A direct consequence of data volume and tool sprawl is an overwhelming flood of alerts directed at IT teams. A significant portion of these alerts are often false positives (over 52%) or redundant notifications (64%) about the same underlying issue. This constant "noise" leads to alert fatigue, where operators become desensitized and may inadvertently ignore or miss genuinely critical alerts. This isn't just an operational annoyance; it represents a substantial business risk. Missing a critical alert due to the surrounding noise can lead to prolonged outages, security breaches, compliance failures, and degraded customer experiences, directly impacting revenue and reputation.
Slow Incident Resolution (MTTR): The combination of data silos, alert noise, and system complexity makes identifying the root cause of problems a slow and arduous manual process. Teams often spend excessive time in "war rooms," sifting through logs and metrics from different tools, attempting to manually correlate events across domains. This significantly increases Mean Time To Detect (MTTD) and Mean Time To Resolution (MTTR), prolonging service disruptions, impacting service level agreements (SLAs), and hindering business continuity.
The table below contrasts key operational metrics under traditional approaches versus those enabled by AIOps, highlighting the tangible improvements achievable.
Metric | Traditional Approach | AIOps-Enabled Approach | Impact |
Alert Volume |
High, Noisy, Uncorrelated |
Significantly Reduced, Contextualized, Prioritized |
Reduced Fatigue, Faster Triage |
Incident Detection |
Reactive, Static Thresholds |
Proactive, Dynamic Anomaly Detection |
Earlier Detection, Outage Prevention |
Root Cause Analysis Time |
Hours/Days, Manual Correlation |
Minutes, Automated Correlation |
Drastically Reduced MTTR |
Remediation |
Manual, Scripted |
Automated Workflows |
Faster Resolution, Reduced Human Error |
Data Utilization |
Siloed, Underutilized |
Unified, Analyzed Holistically |
Better Insights, Optimization |
This stark contrast underscores why organizations are increasingly turning to AIOps to overcome the limitations of traditional IT operations and build more resilient, efficient, and intelligent infrastructure management capabilities.
Inside the AIOps Engine: Core Capabilities Transforming IT
AIOps platforms achieve their transformative potential through a combination of core capabilities that work together to ingest, analyze, and act upon operational data. Understanding these components reveals how AIOps moves beyond simple monitoring to provide intelligent insights and automation:
Data Aggregation & Management: The foundation of any AIOps strategy is the ability to collect and manage vast amounts of data from diverse sources across the entire IT landscape. This includes historical performance data, real-time operational events, system logs, application metrics, network traces, configuration data, infrastructure details, and even IT service management (ITSM) tickets. AIOps platforms must ingest data regardless of its source or format, breaking down the silos created by disparate monitoring tools. Often, this involves establishing a centralized data lake or a unified data layer where information can be consolidated. Crucially, these platforms need real-time processing capabilities to analyze data as it arrives, enabling immediate insights and responses.
AI / Machine Learning Analytics: This is the intelligent core where raw data is transformed into actionable insights. Key analytical techniques include:
Anomaly Detection: Instead of relying on predefined, static thresholds, AIOps uses machine learning algorithms to learn the normal operating behavior of systems and applications over time. It can then identify subtle deviations and statistically significant anomalies that might indicate emerging problems, often before they trigger traditional alerts or impact users. This involves sophisticated pattern recognition capabilities.
Event Correlation: Faced with a storm of alerts from various tools, AIOps applies algorithms, often combined with topology and dependency mapping, to analyze relationships between these events.2 It automatically groups related alerts stemming from a single root cause, suppresses redundant notifications, and filters out noise.4 This drastically reduces alert fatigue and helps teams focus on the real issues.
Root Cause Analysis (RCA): Going a step beyond correlation, AIOps aims to pinpoint the underlying cause of an incident. By analyzing dependencies, contextual data, and the sequence of correlated events, AIOps platforms can identify the likely root cause far more quickly and accurately than manual troubleshooting efforts allow, significantly reducing MTTR. It's important to recognize that correlation groups related symptoms, while RCA identifies the actual disease causing those symptoms – both are valuable, but RCA provides deeper diagnostic power.
Predictive Analytics: More advanced AIOps capabilities leverage historical data and identified patterns to forecast potential future issues. This could involve predicting capacity shortfalls based on usage trends, anticipating component failures, or forecasting performance degradation, enabling truly proactive interventions.
Intelligent Automation & Orchestration: AIOps translates insights into action through automation, reducing manual effort and accelerating responses:
Automated Remediation: Based on identified issues and predefined rules or learned patterns, AIOps can trigger automated workflows or runbooks to execute remediation actions. Examples include restarting a failed service, allocating additional resources, reverting a problematic configuration change, or executing a diagnostic script. This closes the loop from detection to resolution for known issues.
Workflow Orchestration: AIOps platforms can integrate with ITSM tools (like ServiceNow) to automate processes such as creating intelligently enriched incident tickets, prioritizing issues based on business impact, and routing tickets to the appropriate teams.
Visualization/Dashboards: While not an AI function itself, the ability to present complex AIOps insights clearly through intuitive dashboards and visualizations is critical for human operators. Tools like Grafana play a key role in providing a unified view of system health derived from AIOps analysis.
The power of these AI/ML techniques, however, is fundamentally tied to the data they consume. Inaccurate, incomplete, or poorly contextualized data will inevitably lead to flawed analysis, unreliable predictions, and ineffective automation. Therefore, a robust data strategy and mechanisms for ensuring data quality are prerequisites for successful AIOps implementation.
From Insight to Action: Practical AIOps Use Cases & Quick Wins for Infrastructure
While the concept of AIOps can seem broad, its value becomes concrete when examining specific use cases that address common IT infrastructure pain points. Many organizations find success by starting with "quick wins" that deliver immediate value and build confidence before tackling more complex implementations.
Noise Reduction & Intelligent Alerting (Easy Win): This is often the first and most impactful benefit organizations realize. By automatically correlating related events from disparate monitoring tools and suppressing redundant or low-priority alerts, AIOps significantly reduces the sheer volume of notifications hitting operations teams. Furthermore, AIOps enriches the remaining alerts with crucial context – such as topology information, affected business services, historical performance data, or relevant configuration changes – making them immediately more actionable. This directly combats alert fatigue, allowing teams to focus their attention on genuine issues and accelerating initial triage. The immediate relief provided makes this a powerful starting point for demonstrating AIOps value.
Proactive Anomaly Detection & Predictive Maintenance: Moving beyond reactive threshold breaches, AIOps excels at identifying subtle deviations from established normal behavior patterns. This allows for the detection of issues before they escalate to cause user impact or trigger traditional alarms. Practical examples include detecting gradual memory leaks in applications, predicting imminent disk failures based on degrading performance metrics, identifying unusual network traffic patterns that could signal configuration issues or security threats, or spotting performance degradation in specific microservices.
Accelerated Root Cause Analysis (RCA): AIOps dramatically shortens the time required to diagnose the underlying cause of complex problems. Instead of manual log sifting and cross-team troubleshooting sessions that can take hours or days, AIOps platforms analyze correlated event data, topology maps, and configuration changes to automatically pinpoint the likely root cause, often within minutes. For instance, AIOps can rapidly trace a reported application slowdown through dependent services, underlying virtual machines, network paths, and storage systems to identify the bottleneck.
Automated Incident Triage & Initial Remediation Steps (Potential Easy Win): AIOps can streamline the initial stages of incident management. Based on correlated and contextualized alerts, it can automatically generate enriched incident tickets in ITSM platforms, pre-populated with relevant diagnostic information and suggested priority levels. For well-understood, recurring issues with low-risk fixes, AIOps can even trigger automated first-level remediation actions, such as restarting a non-critical service, clearing temporary file systems, or failing over to a redundant component. This frees up L1/L2 support staff for more complex tasks.
Capacity Optimization & Planning: By analyzing historical resource utilization trends (CPU, memory, storage I/O, network bandwidth) and correlating them with performance metrics, AIOps can provide predictive insights into future capacity needs. This helps organizations proactively scale resources to avoid performance bottlenecks, ensure service levels, and optimize infrastructure spending. AIOps can also identify chronically underutilized or over-provisioned resources, particularly in cloud environments, leading to significant cost savings.
Enhanced Security Posture: While AIOps is not a replacement for dedicated security tools like SIEM or SOAR platforms, its ability to detect anomalies in operational data can serve as an early warning system for potential security incidents. Unusual login patterns, unexpected network traffic flows, abnormal resource consumption on specific servers, or deviations in configuration files can all be flagged by AIOps anomaly detection engines.8 Correlating these operational anomalies with alerts from security tools can provide richer context for security investigations, highlighting a growing synergy between IT Operations and Security Operations (SecOps).
Starting with use cases like noise reduction provides immediate relief and builds crucial organizational buy-in. This momentum facilitates the adoption of more advanced capabilities like predictive analytics and automated remediation, driving progressively greater value from the AIOps investment.
The following table summarizes key use cases and aligns them with potential benefits and relevant capabilities offered through Intelligent Visibility and its partners.
Use Case | Description | Key Benefit(s) | Relevant IVI Capability / Partner |
Noise Reduction |
Filtering duplicates, correlating related alerts |
Reduced Alert Fatigue, Faster Triage |
Aegis IR, LogicMonitor, BigPanda |
Anomaly Detection |
Identifying deviations from normal behavior |
Early Issue Detection, Proactive Prevention |
Aegis PM, LogicMonitor, AWS DevOps Guru |
Accelerated RCA |
Automated identification of root causes |
Reduced MTTR, Improved Reliability |
Aegis IR, LogicMonitor, Splunk ITSI |
Automated Remediation |
Triggering automated fixes for known issues |
Faster Resolution, Reduced Manual Effort |
Professional Services (Automation), LogicMonitor, ServiceNow ITOM |
Capacity Optimization |
Predicting resource needs, identifying waste |
Cost Savings, Performance Assurance |
Aegis PM, Professional Services, LogicMonitor, AWS |
Fueling AIOps: The Critical Role of Data Strategy & Observability Pipelines
The success of any AIOps initiative hinges fundamentally on the quality, completeness, and context of the data it consumes. As established, AI and machine learning algorithms require vast amounts of reliable data to learn normal behavior, detect meaningful anomalies, and accurately correlate events. The adage "garbage in, garbage out" is particularly pertinent in the AIOps domain; feeding algorithms with noisy, inconsistent, or irrelevant data will inevitably lead to inaccurate insights, false positives, missed detections, and ultimately, a lack of trust in the system.
Raw telemetry data generated by diverse IT systems rarely arrives in a state ready for optimal AIOps consumption. It is often characterized by:
Noise: Containing irrelevant information or redundant data points.
Lack of Structure: Existing in varied, often unstructured formats (e.g., diverse log formats).
Disparate Schemas: Using different field names and conventions across sources.
Low Value: Including fields or metrics that provide little analytical insight but contribute significantly to volume and cost.
Missing Context: Lacking crucial metadata (e.g., business service impact, user information, deployment details) needed for accurate correlation and prioritization.
Sensitivity: Potentially containing personally identifiable information (PII) or other sensitive data requiring masking or redaction.
Simply forwarding all this raw data directly to AIOps platforms or centralized analytics tools presents significant challenges. It can lead to exorbitant ingestion and storage costs, overwhelm analytical engines, and degrade the quality of ML model training and inference.
This is where the concept of an Observability Pipeline becomes critical. An observability pipeline acts as an intermediary data processing layer, sitting between the data sources (agents, collectors, APIs) and the data destinations (AIOps platforms, monitoring tools, log analytics systems, data lakes). Its primary purpose is to provide control, choice, and optimization over telemetry data streams before they incur costs or are consumed by downstream tools. It effectively decouples data generation from data consumption, offering flexibility and efficiency.
Intelligent Visibility partners with Cribl, a leader in the observability pipeline space, to address these data challenges. Cribl provides a suite of tools (including Cribl Stream, Cribl Edge, and Cribl Cloud) designed to manipulate telemetry data in flight, enabling organizations to:
Route Data Intelligently: Send different subsets of data to the most appropriate destinations based on value and use case. For example, route full-fidelity security logs to a SIEM, summarized metrics to a monitoring platform like LogicMonitor, and sampled trace data to an APM tool, while archiving raw data affordably in object storage.33 This avoids vendor lock-in and optimizes tool usage.
Reduce Data Volume: Filter out noise, drop unnecessary fields, suppress duplicate events, sample high-volume data streams, and aggregate metrics before data is indexed or analyzed, significantly reducing downstream costs without losing critical insights.
Transform and Enrich Data: Normalize data formats and schemas across different sources, parse unstructured logs, mask or redact sensitive information (PII), and enrich events with valuable context (e.g., adding geolocation data based on IP address, tagging events with application or business unit information). This transformation makes data more consistent, compliant, and valuable for AIOps algorithms.
Collect and Replay Data: Efficiently collect data from various sources, including edge devices 34, and provide the ability to replay historical data from low-cost storage (like Amazon S3) back into analytical tools for investigations or model retraining.
By implementing an observability pipeline strategy with tools like Cribl, organizations can directly accelerate and optimize their AIOps initiatives. This approach ensures that AIOps platforms receive cleaner, richer, and more relevant data, leading to more accurate ML models, fewer false positives, faster insights, and significantly lower data processing and storage costs. In essence, an observability pipeline is rapidly becoming not just a helpful addition but a foundational architectural component for achieving scalable, cost-effective, and truly effective AIOps. It directly tackles the data heterogeneity and volume challenges inherent in modern, complex IT environments.
Building an Intelligent Ecosystem: Leveraging Best-of-Breed Technologies
Achieving the full potential of AIOps rarely involves a single, monolithic platform. Instead, a successful strategy typically relies on integrating a curated ecosystem of best-of-breed technologies, each specializing in a particular aspect of data collection, processing, analysis, or action. Intelligent Visibility excels in architecting, implementing, and managing these integrated solutions, leveraging strong partnerships with leading technology providers. This ecosystem approach allows organizations to build a comprehensive AIOps capability tailored to their specific needs.
The partner ecosystem often follows a logical flow, representing distinct layers in the AIOps value chain: Data Generation -> Data Preparation/Optimization -> AIOps Analysis & Core Observability -> Unified Visualization.
Key partners contribute as follows:
LogicMonitor (The AIOps & Observability Hub): Positioned as a central pillar, LogicMonitor's LM Envision platform provides SaaS-based, AI-powered hybrid observability. Recognized as a Visionary by Gartner, LM Envision serves as a core engine for AIOps analysis. Its capabilities include Edwin AI, featuring "Agentic AIOps" for advanced event intelligence, cross-source event correlation (proven to reduce ServiceNow incident volume significantly), root cause analysis, and driving automation. LogicMonitor boasts broad integration capabilities, complimented by Intelligent Visibility's extensive library of custom, optimized, modules for enhanced integration with key infrastructure vendor platforms (Arista, Cisco, Palo Alto, etc.). Its strategic collaborations with AWS (for cloud modernization and migration) and OpenAI (leveraging cutting-edge AI models) signal a commitment to advanced, autonomous capabilities, aligning with the future trajectory of AIOps. Its FedRAMP In Process designation also makes it suitable for public sector applications.
Cribl (The Data Optimizer): As detailed previously, Cribl plays the crucial role of the observability pipeline. It sits upstream from platforms like LogicMonitor, preparing and optimizing telemetry data. By routing, reducing, transforming, and enriching data before it hits the AIOps engine or storage, Cribl ensures higher data quality, controls costs associated with data volume, enhances flexibility, and accelerates the overall AIOps process. Offerings like Cribl Stream, Edge, and Cloud provide deployment flexibility.
AppDynamics: A leading Application Performance Monitoring (APM) solution providing deep visibility into application behavior, transaction tracing, and business context. It integrates tightly with ThousandEyes for end-to-end visibility.
ThousandEyes: Monitors network paths and digital user experience across the internet, SaaS applications, and internal networks. It integrates with AppDynamics and can feed alert data into AIOps platforms (including Cisco Cloud Observability AIOps) via webhooks.
AWS (The Data Source - Cloud & Native Tooling): As a dominant cloud provider, AWS infrastructure and services are critical data sources for AIOps in hybrid environments. Beyond raw infrastructure metrics and logs, AWS offers native observability and AIOps tools:
Amazon CloudWatch: The foundational monitoring service providing metrics, logs, alarms, and basic anomaly detection capabilities for AWS resources and applications.
AWS DevOps Guru: An ML-powered service specifically designed to provide insights and anomaly detection for applications running on AWS, integrating with CloudWatch and aiming to reduce noise and accelerate MTTR for AWS workloads. LogicMonitor's strategic collaboration agreement with AWS further strengthens the integration possibilities, particularly for organizations modernizing their data centers or migrating VMware workloads.
Grafana (The Unified Visualization Layer): Grafana serves as a powerful, open-source platform for visualizing data from the entire AIOps ecosystem. Its strength lies in its ability to connect to diverse data sources – including LogicMonitor, AWS CloudWatch, Prometheus, databases, and many others – and present information in unified, customizable dashboards. This allows operations teams, developers, and business stakeholders to gain tailored views of system health, performance trends, and AIOps-driven insights through interactive graphs, tables, and alerts.
Intelligent Visibility's expertise lies in understanding how these powerful tools interoperate, designing architectures that leverage their respective strengths, and integrating them seamlessly to deliver a cohesive and effective AIOps solution.
Intelligent Visibility's AIOps Advantage: Your Partner in Intelligent Operations
Successfully adopting AIOps is more than just deploying new software; it requires strategic planning, deep technical expertise across multiple domains, effective data management, process integration, and organizational change management. Challenges such as internal skill gaps, resistance to automation, and the complexity of integrating disparate tools can hinder AIOps initiatives. Intelligent Visibility acts as the expert partner to help organizations overcome these hurdles and realize the full benefits of AIOps.
Intelligent Visibility bridges the gap between the potential of advanced AIOps technologies and the practical realities of IT operations. Their value proposition rests on deep expertise, a curated ecosystem of leading partners, and flexible engagement models designed to meet diverse client needs:
Managed AIOps Services (Aegis Suite): For organizations seeking AIOps-driven outcomes without the burden of building and managing the underlying infrastructure, Intelligent Visibility offers its Aegis co-managed services suite. This suite naturally aligns with the core AIOps workflow of Observe -> Engage -> Act:
Aegis PM (Performance Monitoring): This service provides the foundational 'Observe' layer. Leveraging powerful observability platforms like LogicMonitor, Aegis PM delivers full-stack visibility across networks, infrastructure, cloud, and applications, collecting the high-quality, comprehensive data essential for effective AIOps.3 It forms the data bedrock upon which intelligent analysis is built.
Aegis IR (Incident Response): This service embodies the 'Engage' and 'Act' phases. Aegis IR utilizes the insights generated by AIOps tools – such as event correlation, anomaly detection, and root cause analysis derived from platforms like LogicMonitor's Edwin AI – to enable faster, more accurate incident detection, triage, and remediation. This directly translates to reduced Mean Time To Resolution (MTTR) and improved service availability. While Aegis PM and IR are central to AIOps, insights derived from the platform can also inform other managed services like Aegis CM (Configuration Management) and Aegis LM (Lifecycle Management), ensuring changes are validated against performance data and lifecycle activities are prioritized based on operational risk or impact.
Building AIOps Capabilities (Professional Services): For clients who prefer to develop and own their internal AIOps capabilities, Intelligent Visibility offers comprehensive professional services. Their team provides expert guidance throughout the AIOps journey:
Strategy & Design: Assisting clients in defining clear AIOps objectives, assessing their current operational maturity and data readiness, selecting the optimal combination of tools from partners like LogicMonitor, Cribl, Cisco, AWS, and Grafana, and designing a robust, scalable AIOps architecture.
Implementation & Integration: Deploying the chosen platforms, configuring data ingestion and processing pipelines using tools like Cribl, setting up monitoring and AIOps rules within LogicMonitor, integrating data feeds from sources like Cisco infrastructure and AWS CloudWatch, and building unified visualization dashboards in Grafana. This leverages Intelligent Visibility's strong capabilities in infrastructure automation.
Customization & Optimization: Tailoring AIOps algorithms, correlation rules, automation workflows, and reporting dashboards to meet the unique requirements and operating context of the client's environment.
Crucially, Intelligent Visibility focuses on delivering tangible business outcomes – improved system reliability and uptime, increased operational efficiency, optimized resource utilization and cost savings, enhanced security posture, and ultimately, better user and customer experiences – rather than simply deploying technology for its own sake. Their expertise ensures that the sophisticated capabilities of the partner ecosystem are translated into measurable improvements for the client's business.
As robust observability is the prerequisite for effective AIOps, further details on establishing this foundation can be found on Intelligent Visibility's Network and Infrastructure Observability page.
Looking Ahead: The Trajectory Towards Autonomous Operations
AIOps is not a static endpoint but rather an evolving journey towards increasingly intelligent and autonomous IT operations. The trajectory moves progressively from enhancing human capabilities to automating more complex decision-making and actions:
Reactive: Traditional monitoring based on static thresholds and manual responses.
Proactive: Using anomaly detection to identify potential issues before they cause impact.
Predictive: Leveraging historical data and ML to forecast future events like capacity shortfalls or failures.
Autonomous: Systems capable of self-diagnosis, self-healing, and self-optimization with minimal human intervention.
The emergence of concepts like "Agentic AIOps," as highlighted by partners like LogicMonitor, signifies a significant step towards this autonomous future. Agentic systems aim to go beyond simply identifying problems and suggesting solutions; they are designed to autonomously decide and execute the appropriate remediation actions, potentially adapting to novel situations without relying solely on pre-programmed rules. This represents a potential paradigm shift, moving closer to the long-held vision of autonomic computing.
However, achieving true autonomy requires overcoming significant technical and organizational challenges. Building trust in AI-driven decisions, ensuring the safety and reliability of automated actions, and establishing appropriate human oversight mechanisms ("human checkpoints") are critical considerations. While the technology is advancing rapidly, the adoption of fully autonomous remediation for critical systems will likely be gradual, requiring careful validation and governance.
Another key trend is the increasing alignment of AIOps with business outcomes. Future platforms will likely become more adept at correlating IT operational metrics (like latency, error rates, resource utilization) directly with key business performance indicators (KPIs) such as revenue, customer conversion rates, or transaction volumes. This will provide clearer visibility into how infrastructure health and performance directly impact the business's bottom line, enabling more informed decision-making regarding IT investments and priorities.
Intelligent Visibility, through its expertise and partnerships with forward-looking vendors like LogicMonitor, is well-positioned to guide clients along this evolutionary path. They can help organizations leverage current AIOps capabilities effectively while preparing for the next generation of more autonomous and business-aware operational intelligence.
Conclusion: Embark on Your AIOps Journey with Confidence
The escalating complexity of modern IT infrastructure, coupled with relentless business demands for performance, reliability, and agility, has rendered traditional operational approaches inadequate. AIOps has emerged not as a futuristic buzzword, but as a necessary evolution, offering a path to transform reactive IT operations into proactive, predictive, and automated engines that drive business value. By leveraging AI and machine learning to analyze vast data streams, AIOps delivers tangible benefits: drastically reduced alert noise, faster incident resolution (MTTR), proactive issue prevention, optimized resource utilization, and ultimately, more resilient and efficient IT services.
Successfully navigating the AIOps landscape, however, requires more than just technology. It demands a strategic approach, deep technical expertise, robust data management practices, and often, a trusted partner to guide the journey.
Intelligent Visibility stands uniquely positioned to be that partner. With proven expertise in modern IT infrastructure, observability, and automation, coupled with a carefully curated ecosystem of leading technology partners – including LogicMonitor for AI-powered observability, Cribl for data optimization, Cisco and AWS for foundational data sources, and Grafana for unified visualization – Intelligent Visibility offers a comprehensive approach to AIOps.
Whether an organization seeks to offload operational burdens through the Aegis Managed Services suite, gaining AIOps-driven outcomes like enhanced performance monitoring (Aegis PM) and rapid incident response (Aegis IR), or prefers to build internal capabilities with expert guidance via Intelligent Visibility's Professional Services, a tailored path exists. Intelligent Visibility focuses on understanding specific challenges and delivering measurable business outcomes, ensuring that AIOps investments translate into real-world improvements in reliability, efficiency, and user experience.
Ready to move beyond the noise and harness the power of AIOps for your IT infrastructure?
Contact Intelligent Visibility today for an AIOps readiness assessment to understand how these capabilities can address your specific challenges.
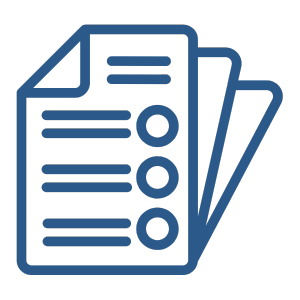
Unified Infrastructure Management Fabric - Introduction
Discover how Intelligent Visibility’s Intelligent Operations platform unifies infrastructure observability and AIOps to deliver end-to-end visibility, faster issue resolution, and proactive IT operations — turning complexity into competitive advantage.
Read Now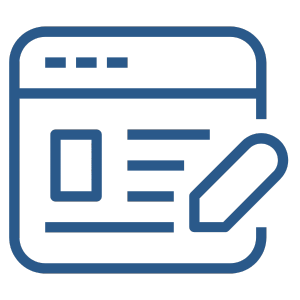
Blog - Observability for Hybrid Infrastructure
Learn how cloud-native observability and hybrid UIMF boost visibility, performance, and agility across hybrid and multi-cloud environments.
Read Now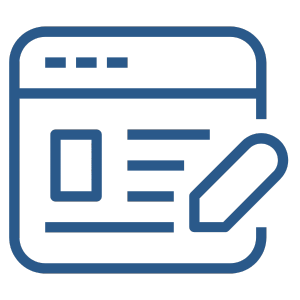
Blog - Importance of API-first Infrastructure
See how an API-first infrastructure mindset with UIMF drives automation, agility, and seamless integration across hybrid environments.
Read More